Research shows a significant increasing trend of institutional investors in the last fifteen years, directing funds toward ESG-related investments and funds. In 2005, institutional investors held $1.5 trillion in ESG-based funds, while by 2020, the level of ESG-based assets quadrupled to reach $6.2 trillion. This trend is a solid indicator of the need to develop reliable tools to distinguish between the companies and funds that are genuinely ESG-related and the companies involved in “Greenwashing.” We believe that the trend shown in the Figure below will continue to rise even faster if investors can determine whether a company or a fund is taking steps to improve its ESG profile with a high level of confidence.
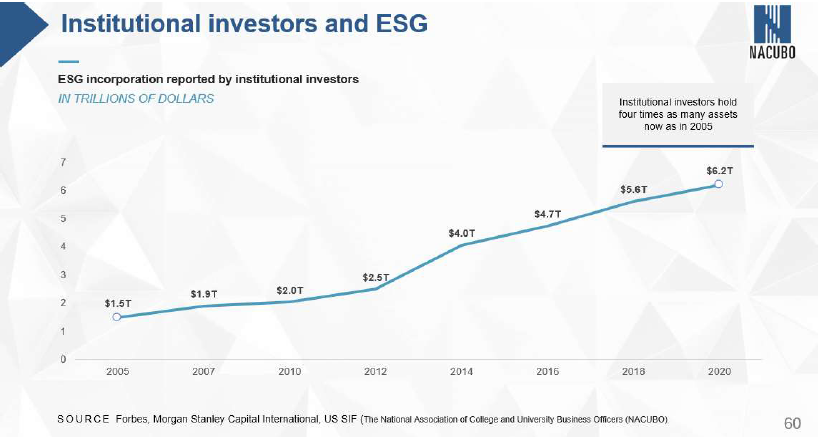
and funds (Source: Forbes, Morgan Stanley Capital International, US SIF
(The National Association of College and University Business Officers
(NACUBO))
The challenges with ESG Data also create opportunities for interdisciplinary approaches to developing and offering tools for disentangling the information from the noise in reporting ESG metrics and unraveling the truth about corporate dedication and actions towards becoming more socially responsible. The newly developed methodologies could measure the “environmental legitimacy” of the corporations and offer evidence of “Greenwashing,” a term describing companies that are “talking the talk, but not walking the walk.”
To provide a good understanding of Greenwashing, we need to collect and analyze many data, including market research and specific company data. We consider different data sources combining specific corporate news articles, company filings, and alternative data sources. The main challenge of data gathering is that often high-quality data sources are proprietary and inaccessible.
Hence, we focus on publicly available data from reputable data sources. One of the most valuable data sources in the U.S. is the SEC’s Electronic Data Gathering, Analysis, and Retrieval, which contains all company filings of publicly-traded companies on the stock exchanges. Using these documents, we can identify what the company reports and how they view themselves regarding any ESG issue.
Once we have one side of the story, we need data from the other; let’s call this side the media side. We intend to gather corporate news articles from various media websites, such as GDELT, Google RSS services, and Yahoo Finance news. We also consider social media portals such as Reddit and Twitter as supplementary data. These two platforms offer an API that gives access to their content, and they are accessible under certain limits. We also use APIs like Yahoo finance which provide financial information about a company like stock prices, trading volumes, and ESG risk scores. Before offering any machine learning, Artificial Intelligence, or other technological solution in finance, a crucial part of our research is to understand the regulatory environment and the repercussions of technology-based solutions within the securities and investment regulatory framework. Another important aspect is finding definitions and rules for determining ESG-related metrics as benchmarks for company ESG rankings. The European Union and the United States have already issued guidelines about greenhouse gas emissions, carbon neutralization diversity, etc. These documents offered recommendations about various aspects related to ESG, explaining considerations for improving corporate ESG rating, and outlining sanctions for the company’s negative impacts on specific aspects of ESG. One of the challenges in tackling the ESG issue and determining whether companies’ trajectory is improving, or deteriorating is to define a taxonomy of words that will represent the main topics of our environmental, social, and corporate governance. We establish a list of words and phrases that can be used when searching for meaningful news articles and social media posts and through the regulatory mandated corporate disclosure documents. We finally connect the search terms and phrases with selected corporations to extract information such as the frequency of co-occurrence between the company names and a set of ESG terms. We then map out the sentiments for the extracted text to understand the relationship between ESG-related topics and companies. This information gives us a timestamp with frequencies and sentiments where we observe the company’s good or bad ESG decisions. We could then overlap the frequency and sentiment graphs with the stock price return information to relate the ESG (qualitative) and the price (quantitative) indicators of company performance.