When considering ESG investments, portfolio managers look into companies or funds with high ESG ratings. Several companies, including MSCI, S&P, Sustainalytics, CDP, ISS, Bloomberg, and others, provide ESG ratings. However, the consensus level regarding the ratings is relatively low, which makes ESG-focused investments challenging. For example, let’s look at the correlations of ESG Ratings across different providers. We observe a correlation of 7% between ISS and CDP ratings, 16% between MSCI and CDP, 58% between Sustainalytics and Bloomberg, and the highest correlation of approximately 74% between Bloomberg and S&P. This dispersion among ESG ratings from different data providers is confusing for investors. The discrepancies observed in the Figure below occur because many of the ESG measures are subjective, disclosure can be inconsistent, there may be biases in the data, and there could be changes in corporate behavior.
Existing solutions from technology-based providers offer insights into how companies can improve their ESG index or score and get a better overall view of their index of sustainability. The insights are generated by building a specific model for the company based on accessing, organizing, and analyzing relevant company files. Another interdisciplinary, analytical solution to increasing the ESG transparency is to provide datasets of raw ESG indicators. This solution involves collecting company data from various sources, such as company documents, news, social media, and alternative data sources. The data can then be used either in a row format or in a more insightful way based on collaborative work between data scientists and high-level investor knowledge of market insights and company ESG involvement. This model offers excellent synergetic and profitable cooperation for influencing corporate behavior and contributing to enhanced sustainability and financial stability.
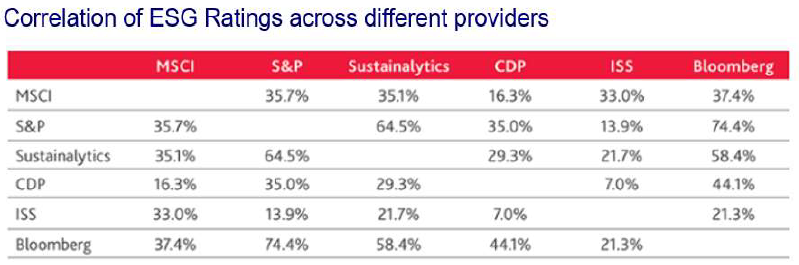
CDP, ISS, and Bloomberg, where we observe a high level of dispersion.
Machine learning and AI-based solutions can contribute to the meaningful representation of each company’s most critical ESG factors, emphasizing corporate dedication to ESG issues by creating dashboards showing what the company reports and what the company does about ESG. The dashboard would extract and visualize the overall picture of a company’s ESG involvement. The insight is generated by collecting every data point for the company from internal and external sources and giving more profound insight into the company sustainability index. The AI-based solution cold model dynamic changes and go beyond ESG reporting by providing a future estimated outlook into corporate ESG risks. On the other hand, the AI-based solution could offer positive impacts indices and explainable results from machine learning analysis and extract insights about how social and environmental trends will impact the company. A similar study could offer insights into how negative scenarios (e.g., climate or social disasters) will affect the company’s future and determine its resilience to adverse events.